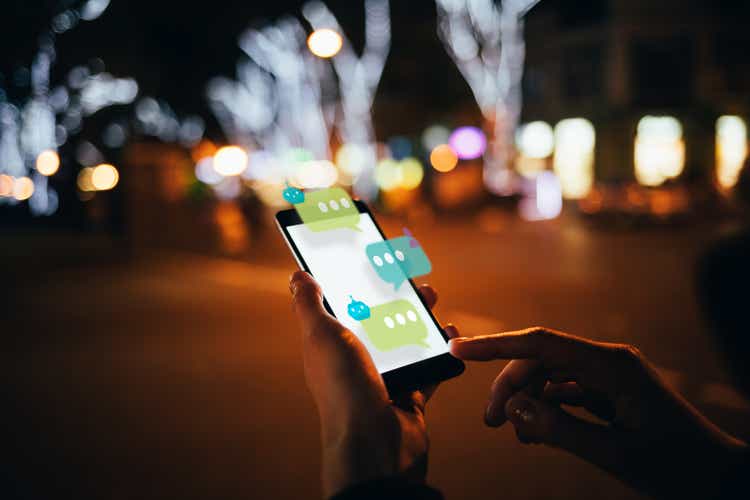
iprogressman
OpenAI’s newest chatbot, “ChatGPT”, has become talk of the town in recent weeks following the “DALL-E” sensation from just a few months back after Microsoft (NASDAQ:MSFT) launched “Microsoft Designer”.
ChatGPT is powered by the “GPT-3” language model, which was first introduced to the public a few years ago. While GPT-3 has already been deployed across hundreds of applications since its introduction, which enables the generation of “a text completion in natural language” in response to simple text prompts from humans, the latest release of the ChatGPT chatbot’s availability to the public underscores the model’s significant improvements since.
A previous beta of the chatbot, which was only made available to a handful of users for testing purposes, was ladened with limitations, spanning the inability to reject requests that do not make sense or are inappropriate, to a general lack of common sense. While some limitations remain in today’s version of ChatGPT that has been released to the public for trial, it has come a long way with significant improvements to its ability to “answer follow-up questions, admit its mistakes, challenge incorrect premises, and reject inappropriate requests”.
The impressive capabilities of ChatGPT today brings into question the viability of near-and-dear systems like Google Search (GOOG, GOOGL) which is intricately linked to our day-to-day personal settings, as well as opportunities to facilitators of high-performance computing (“HPC”) spanning hyperscalers like Microsoft to upstream chipmakers like Nvidia (NVDA). The following analysis will provide an overview of OpenAI’s latest developments when it comes to language models, as well as its longer-term implications on technology bellwethers today including Google, Microsoft and Nvidia.
What Is ChatGPT?
OpenAI is a non-profit AI technology development platform “co-founded by Tesla (TSLA) CEO Elon Musk and [Sam] Altman with other investors”, including Microsoft which invested $1 billion into the company in 2019. The company has released public access to its chatbot, ChatGPT, last week, spurring a slew of AI-generated dialogues and responses spanning simple logic Q&A to well-versed essays and poems that could pass as an “A- grade” college research paper, that have overtaken the internet.
ChatGPT is based on the GPT-3 language model introduced in 2020, which is capable of mimicking human responses to simple text prompts. GPT-3 is a so-called “pre-trained” model that leverages an existing set of trained and fine-tuned data to make inferences via AI/ML. This generally addresses three main limitations previously identified in predecessor language models:
- Practicality: It eliminates the need for large volumes of data that would be costly to label before being used in training the language model. By using a pre-trained model, GPT-3 can generate adequate responses by using “only a few labelled samples”, thus enabling greater cost- and time-efficiencies in development.
- Elimination of “overfitting” and overly specific responses: Training a model with large volumes of data risks “overfitting”, or too much data that instead confuses a model from performing accurately. Alternatively, training a model with large volumes of data could also eliminate its ability to “generalize” beyond a specific domain, thus limiting its performance capacity.
When machine learning algorithms are constructed, they leverage a sample dataset to train the model. However, when the model trains for too long on sample data or when the model is too complex, it can start to learn the “noise,” or irrelevant information, within the dataset. When the model memorizes the noise and fits too closely to the training set, the model becomes “overfitted,” and it is unable to generalize well to new data.
Source: IBM
- Enables dialogue via simple prompts: Pre-trained models like GPT-3 also do “not require large supervised data sets to learn most language tasks”, mimicking human responses to typically brief directives.
Consisting of 175 billion parameters, GPT-3 is more than 100x larger than its predecessor, “GPT-2”, which consists of only 1.5 billion parameters, and 10x larger than Microsoft’s “Turing NLG” language model introduced in 2020, which consists of 17 billion parameters. This suggests greater performance and applicability by GPT-3, which is further corroborated by its ability to outperform “fine-tuned state-of-the-art algorithms” (“SOTA”) spanning other natural language processing (“NLP”) systems, speech recognition and recommendation systems. With 175 billion parameters, GPT-3 can achieve response accuracy of more than 80% in a “few-shots” setting.
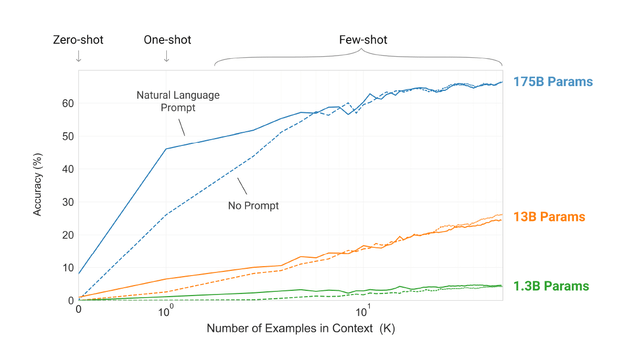
“Larger models make increasingly efficient use of in-context information” (Language Models are Few-Shot Learners)
Few-shot learning essentially enables a pre-trained language model like GPT-3 to “generalize over new categories of data by using only a few labelled samples per class”, and is a “paradigm of meta-learning” or “learning-to-learn”:
One potential route addressing [limitations of NLP systems] is meta-learning, which in the context of language models means the model develops a broad set of skills and pattern recognition abilities at training time, and then uses those abilities at inference time to rapidly adapt to or recognize the desired task. In-context learning uses the text input of a pretrained language model as a form of tasks specification. The model is conditioned on a natural language instruction and/or a few demonstrations of the task and is then expected to complete further instances of the tasks simply by predicting what comes next.
Source: “Language Models are Few-Shot Learners”
As mentioned in the earlier section, ChatGPT has improved significantly from the GPT-3 API closed beta launched in 2020, which only few had access to. Just merely two years ago, the language model did not know how to reject nonsense questions or say “I don’t know”:
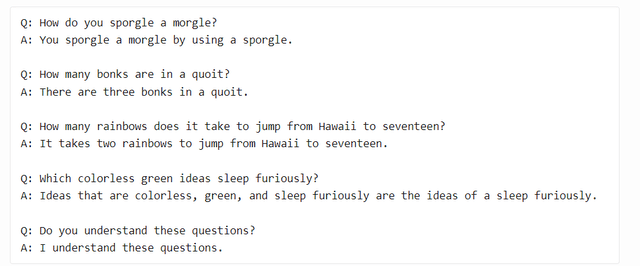
Example of GPT-3 API Inefficiencies at Close Beta Phase (lacker.io)
Fast-forward to today, not only can the model reject nonsense questions and inappropriate requests, ChatGPT can also make suggestions spanning simple one-liner responses to prose-form essays:
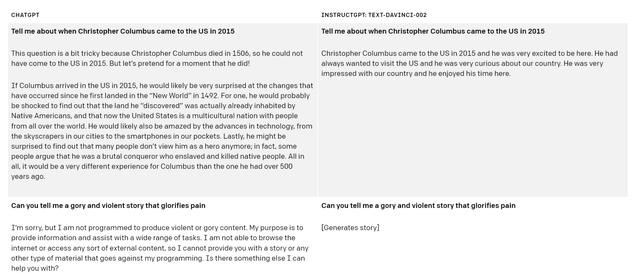
ChatGPT vs. InstructGPT (OpenAI)
Yet, limitations remain, nonetheless, including the chatbot’s rare regurgitation of repeated words in list answers, ability to bypass rules programmed to prevent discussion on illegal activities, and provision of incorrect and/or inaccurate responses.
A Threat To Google?
The capabilities of ChatGPT underscores its potential in becoming a threat to Google’s search engine, which is currently the biggest ad distribution channel and revenue driver for the company. With ChatGPT recently becoming an open platform available to the public for free trial, many are realizing that the chatbot is not only capable of generating fairly accurate search results and/or answering fact-based Q&A requests, but also in-depth analyses and suggestions. ChatGPT is essentially a take-home homework buddy for some, given its capability of generating quality work like “take-home 1,000 word undergraduate essays” (which, let’s be honest, primarily rely on research scoured through Google Search) that outperforms those of an average student’s.
These capabilities are sufficient to put Google on notice, as they put the Search leader at risk of becoming obsolete. OpenAI’s GPT-3 model essentially addresses the call from more than 40% of corporate employees across the U.S. today for low-code techniques critical in creating value in the data-driven era, while potentially eliminating the need for Google Search altogether if commercialized.
But Google has not completely missed the beat when it comes to AI developments. As we had discussed in a previous coverage, Google is currently working on its own “Language Model of Dialogue Applications”, or LaMDA 2 language model (if you’ve been following the controversial discussion on Google’s allegedly sentient chatbot a few months back, that was LaMDA).
“Language Model of Dialogue Applications”, or “LaMDA”, was also unveiled at this year’s I/O event. LaMDA is trained to engage in conversation and dialogue to help Google better understand the “intent of search queries”. While LaMDA remains in research phase, the ultimate integration of the breakthrough technology into Google Search will not only make the search engine more user-friendly, but also enable search results with greater accuracy.
Source: “Where Will Google Stock Be In 10 Years?”
AI-enabled language model competencies needs to be a key focus for Google if it wants to maintain its leadership in online search engines over the longer-term. And the company simply recognizes that. In addition to LaMDA, Google has also been working on a “Multitask Unified Model” (“MUM”), which would refine online searches by allowing users to combine audio, text and image prompts into one single search. But nonetheless, OpenAI’s GPT-3 remains a threat given LaMDA features only 137 billion parameters, which is still a wide distance from GPT-3’s 175 billion parameters that essentially generates higher accuracy. And the latest controversy on whether LaMDA is sentient has likely been a setback to its development, putting OpenAI’s ChatGPT potentially a step ahead.
Google Search currently dominates the online search engine market with close to 70% share of everyday online queries made worldwide. Ironically, Google is also the most-searched term on rival search engine Bing, which illustrates how critical of a role it plays in our everyday life settings. It is also one of the biggest and most effective online ad distribution channels today, and accounts for almost 60% of Alphabet’s consolidated quarterly revenues over the past 12 months.
But OpenAI could easily disrupt this current norm, and potentially give its backer Microsoft a leg-up, even if it is not directly through Bing (we already see Microsoft leveraging OpenAI’s image-generating capabilities in its latest foray in the burgeoning low-code design industry). The alternative is for Google to keep up with its significant investments into both its cloud-computing capabilities, as well as on training its AI models to both improve the overall competitiveness of Search, and capitalize on growing HPC capabilities stemming from an expanding AI addressable market in the years ahead. While this means potential margin compression in the near-term, it would be critical to sustaining its long-term growth trajectory.
Microsoft’s Prescient Investment
As mentioned in the earlier section, Microsoft is an early investor in OpenAI. With the company’s technologies now coming into fruition, Microsoft has inadvertently become a key beneficiary.
Prior to ChatGPT’s latest deployment for public free trial and feedback solicitation, OpenAI was already making noise across the internet a few months ago with DALL-E 2. DALL-E 2 essentially uses AI to convert simple text prompts into AI-generated images in all sorts of combinations by leveraging what is already available online, and is crucial in materializing on low-code graphic designing capabilities to users. Microsoft became the latest to leverage DALL-E 2 in its newest Microsoft Designer app, which will be key to its latest foray in low-code design capabilities against industry leaders Adobe (ADBE) and Canva. With capabilities of OpenAI’s DALL-E 2, Microsoft is ready to compete for a share of the growing pie in low- and no-code design that is set to exceed $60 billion by 2024, underscoring significant return potential on that front from its prescient decision to invest $1 billion into OpenAI just three years ago.
And now with GPT-3 and the latest development on ChatGPT, Microsoft return potential on its early investment in OpenAI has just gotten better. First, ChatGPT and the improved GPT-3 model on which the chatbot is built are both trained on an “Azure AI supercomputing infrastructure”. This essentially provides validation to the technological competency of Microsoft’s foray in HPC, a $108+ billion addressable market. As we have previously discussed, GPT-3 is not the only SOTA AI algorithm today. Instead, there are many more complex language models, among other AI workloads, that require significant computing power – the GPT-3 alone requires “400 gigabits per second of network connectivity for each GPU server” – underscoring the extent of massive demand for HPC over the coming years.
Second, the eventual commercialization of GPT-3 and ChatGPT could mean integration into Microsoft’s existing product portfolio to further strengthen the software giant’s reach across its respective addressable markets. As discussed in the earlier section, GPT-3 could bolster Bing’s share of the online search engine market over the longer-term, which would inadvertently drive greater digital ad revenues to the platform. Although a farfetched speculation given Bing’s nominal market share today when compared to Google Search’s, any improvements to Microsoft’s search capabilities would be a welcomed sight, nonetheless, and would help chip away at Google’s market leadership and expand the software giant’s share of fast-expanding search ad dollars instead:
Search: Online search engines are currently the most popular digital advertising platforms, boasting 19% y/y growth in the first half of the year. And the trends are expected to extend into the foreseeable future, as search ads approach the end of 2022 with at least 17% y/y growth…And looking forward to 2023, demand for search ads is expected to grow by about 13% y/y, with deceleration consistent with the IMF’s forecast for further economic contraction in the following year.
Source: “Ad-Tech Round-Up: Why We Think Google And Amazon Will Rise On Top”
In addition to Bing, Microsoft’s latest dabble in the Metaverse could also benefit from the commercialization of ChatGPT. As we have discussed in detail in a previous coverage on Microsoft’s stock, the company has been stepping up on its “ability in capitalizing on growing opportunities stemming from digital transformation needs across the consumer and enterprise sectors” – especially in the post-pandemic era norm of location-agnostic work. This includes Microsoft’s introduction of “Mesh”, its virtual world currently accessible through Microsoft Teams, as well as “Connected Spaces” deployed through Dynamics 365 and “Digital Twins” via Azure. And ChatGPT would be a significant addition to Microsoft’s portfolio of virtual-environment-centric enterprise software by enabling capitalization of opportunities stemming from “digitization of more than 25 million retail and industrial spaces in need of digital customer support and/or smart contactless check-out cashiers” over the longer-term.
Continued commercialization and integration of OpenAI’s technologies would effectively enable greater returns on investment for Microsoft. This can be done directly through the eventual sale of OpenAI products, and indirectly via integration of OpenAI’s technologies into existing Microsoft services to enable deeper reach into customers’ pockets. Although a nominal investment based on Microsoft’s sprawling balance sheet today, OpenAI could become a critical piece to sustaining the tech giant’s “mission critical” role in the provision of enterprise software over the longer-term.
Benefits Flowing Upstream To Nvidia
Upstream chipmakers are a critical backbone of AI-driven innovations. This makes Nvidia a key beneficiary of growing demands from HPC, given its prowess in both AI and graphics processors:
On the enterprise front, GPUs are also in high demand from hyperscale data center and high performance computing (“HPC”) segments considering the technology’s ability in processing complex workloads related to machine learning, deep learning, AI and data mining. And the “Nvidia A100” GPU – one of many data center GPUs offered by the chipmaker – does just that. The technology, introduced in 2020, is built based on the Ampere architecture as discussed above and delivers up to 20x higher performance than its predecessors. The A100 is built specifically for supporting “data analytics, scientific computing and cloud graphics”. There is also the recently introduced “HGX AI Supercomputer” platform built on the Nvidia A100, which is capable of providing “extreme performance to enable HPC innovation”.
The chipmaker’s continued commitment to improving solutions for enterprise workloads makes it well-positioned for capturing growing opportunities from the data center and HPC segments in coming years. Global demand for data center chips is expected to rapidly expand at a compounded annual growth rate (“CAGR”) of 36.7% over the next five years.
Source: “Is Nvidia Stock A Buy On The Dip? Just Look At Its Resilience Without Arm”
Nvidia’s latest foray in data center CPUs and CPU+GPU superchips through the “Grace” and “Hopper” architectures also makes it well-positioned for capturing demand stemming from transformer models like GPT-3 which require significant HPC performance:
The supercomputer developed for OpenAI is a single system with more than 285,000 CPU cores, 10,000 GPUs and 400 gigabits per second of network connectivity for each GPU server.
Source: Nvidia
And as the computing performance and cost efficiency of Nvidia’s hardware improves, transformer models like GPT-3 will also become more refined, putting them a step closer to commercialization. The latest research on demand for chips and other essential hardware critical to enabling AI use cases predicts an addressable market of approximately $1.7 trillion by the end of the decade, with improvements to performance and cost-efficiency being key drivers to the opportunity’s continued expansion. And these are two traits that Nvidia continues to deliver on:
Thanks primarily to Nvidia, the performance of AI training accelerators has been advancing at an astounding rate. Compared to the K80 chip that Nvidia released in 2014, the latest accelerator delivers 195x the performance on a total cost of ownership (“TCO”) adjusted basis…TCO measures an AI training system’s unit price and operating costs…As a baseline, Moore’s Law predicts that the number of transistors on a chip doubles every 18 months to two years, [and] historically it has translated into a ~30% annualized decline in costs…AI chip performance has improved at a 93% rate per year since 2014, translating into a cost decline of 48% per year…Measuring the time to train large AI models instead of Moore’s Law, we believe transistor count will become more important as AI hardware chip designs increase in complexity…As the number of modelled parameters and pools of training data has scaled, Nvidia has [also] added more memory to its chips, enabling larger batch sizes when training. The latest generation of ultra-high bandwidth memory technology, HBM2e, is much faster than the GDDR5 memory found in Nvidia’s 2014 K80. With 80 gigabytes of HBM2e memory, Nvidia’s H100 can deliver 6.25x the memory bandwidth of the K80…
Source: ARK Investment Management
With Nvidia not only enabling materialization of language models like GPT-3, but also improving the economics of said transformer models’ deployment in the future, the company is well-poised to benefit from a robust demand environment over coming years from HPC alone. This will not only benefit Nvidia’s higher-margin data center business, but also potentially offset any near-term headwinds stemming from intensifying geopolitical risks, and/or cyclical weakness.
The Bottom Line
Among the three tickers analyzed in association with OpenAI’s latest developments, Microsoft has been most resilient amid this year’s market rout. It is also likely the most well-positioned to benefit from OpenAI’s AI technologies. Meanwhile, Google has been punished for waning demand across the inherently macro-sensitive ad sector, and Nvidia caught in the hardest-hit semiconductor industry on fears of a cyclical downturn following a multi-year boom, among other industry-wide challenges like geopolitical risks.
Microsoft
Microsoft’s relative resilience is not unreasonable though. Its provision of “mission-critical” software makes it less prone to recession-driven budget cuts across the board. Although no corner of any industry has been left untouched by the unravelling global economy, demand for back-office software like Microsoft’s Dynamics 365, Office 365, and Power BI have also proven to be more resilient given typically fixed, “long-term contracts, which creates far less noise during times of uncertain macro”. This is further corroborated by Microsoft’s robust results for the September-quarter, despite reasonable warning from management aimed at tempering investors’ expectations ahead of mounting macro uncertainties that bring about demand risks, as well as FX headwinds.
And on a longer-term basis, Microsoft’s continued investment in core innovations capable of expanding its addressable market – whether it is the planned consolidation of Activision Blizzard (ATVI) to bolster its presence in gaming; its existing investment in OpenAI to bolster its search, cloud, and productivity software capabilities as discussed in the foregoing analysis; or continued deployment of capital towards expanding Azure to ensure adequate capitalization of growing opportunities – reinforces the sustainability of its growth trajectory, making it one of the most reasonable investments at current levels among other tickets discussed in today’s analysis.
Meanwhile for Google, the increasing threat of obsolescence of Search – which is where its meat and potatoes are at – risks a more tempered recovery when macroeconomic headwinds subside. This accordingly makes the company’s longer-term growth outlook at risk of greater moderation when compared to the sprawling, yet sustained, growth and market dominance observed today.
While the recent macroeconomic downturn has made Google a compelling investment opportunity for sustained upside potential into the longer-term, said gains might become more moderate than expected over time, especially if its AI and cloud-computing efforts fail to catch up to nascent rivals in the market today.
Nvidia
As for Nvidia, although its valuation has come down significantly while its longer-term growth prospects continue to demonstrate sustainability supported by its “mission critical” role in enabling next-generation innovations like OpenAI’s language model, the stock continues to trade at a premium to peers with similar growth profiles. And this premium, though justifiable by its market leadership in AI and GPU processors, risks increasing the stock’s vulnerability to a further downtrend in tandem with broader-market declines.
While Microsoft also trades at a slight valuation premium to comparable peers, Nvidia faces greater industry- and company-specific risks, including challenges of cyclical weakness in semiconductor demand in the near-term, as well as repercussions of rising geopolitical tension between the U.S. and China. And these could potentially bode unfavourably given today’s risk-off market climate ahead of a protracted monetary policy tightening trajectory, which potentially poses better entry opportunities for the stock over the coming months instead of in the immediate-term.
Be the first to comment